important to understanding data because they allow people to make inferences about a population from a sample. This blog post discusses 5 examples of inferential statistics, including their definitions, uses, types, advantages and disadvantages. Whatever your background in statistics - student, professional or just interested in learning more - this guide is very informative.
Inferential Statistics: What is it?
A sub-branch of statistics known as inferential statistics allows us to make general inferences and conclusions about a population. From a sample of data obtained from that community. Unlike descriptive statistics that show the available data, inferential statistics help us make decisions that go beyond the immediate data.
For example, if a researcher wants to know the average height of adult males in a country, he or she can measure the height of a random sample of males and use inferential statistics to determine the average height of all men in that country.
Why use model statistics?
Statistics should be used appropriately in many contexts for several reasons:
- Generalizability: It allows researchers to generalize findings from a sample to the population.Most of the time the measurement is correct.
- Intelligence: Businesses and decision makers use statistical analysis to make decisions based on data analysis.
- Hypothesis Testing: They help test hypotheses and determine the validity of hypotheses.
- Predictive analytics:Statistics can predict future results based on current results.
Types of inferential statistics
Inferential statistics can be divided into two main categories:
1. Confidence intervals
Confidence intervals are a typical example of inferential statistics.They provide a range of possible values for a true population parameter. For example, if a researcher wants to estimate the average height of adult males in a city, he or she can measure a sample of males and calculate a confidence interval. If the interval is between 175 cm and 180 cm with a 95% confidence level, the researcher is 95% certain that the average height of all the people in the town is within this range.
2. Hypothesis testing
Hypothesis testing is another important part of statistical analysis. Researchers often create a null hypothesis (H0) and an alternative hypothesis (H1) to test a claim. For example, a company may want to test whether a new marketing strategy will increase sales. By analyzing the patterns in the sales data, the company can use statistical tests (such as t-tests and chi-square tests) to determine whether there is enough evidence to rule out the false accusation. . This supports the alternative hypothesis
3. Regression analysis
Regression analysis is widely used in inferential statistics to understand the relationship between variables. For example, a researcher may want to examine the relationship between advertising costs and sales revenue. Using regression analysis, they can determine whether increased advertising spending will increase sales and can predict future sales based on different levels of advertising.
4. Analysis of Variance (ANOVA)
ANOVA is a statistical method used to compare means between three or more groups. For example, the researcher wants to examine the effectiveness of different teaching methods. By conducting an ANOVA test, they can determine if test results differ between groups using different learning methods, so they can draw conclusions about the method.
5. Quadratic test
The chi-square test is used in statistical modeling to evaluate relationships between independent variables. For example, a researcher wants to investigate whether there is a relationship between gender and gender. By collecting sample data and performing a chi-square test, they can assess whether the observed voting patterns differ across genders, providing insight into voter behavior.
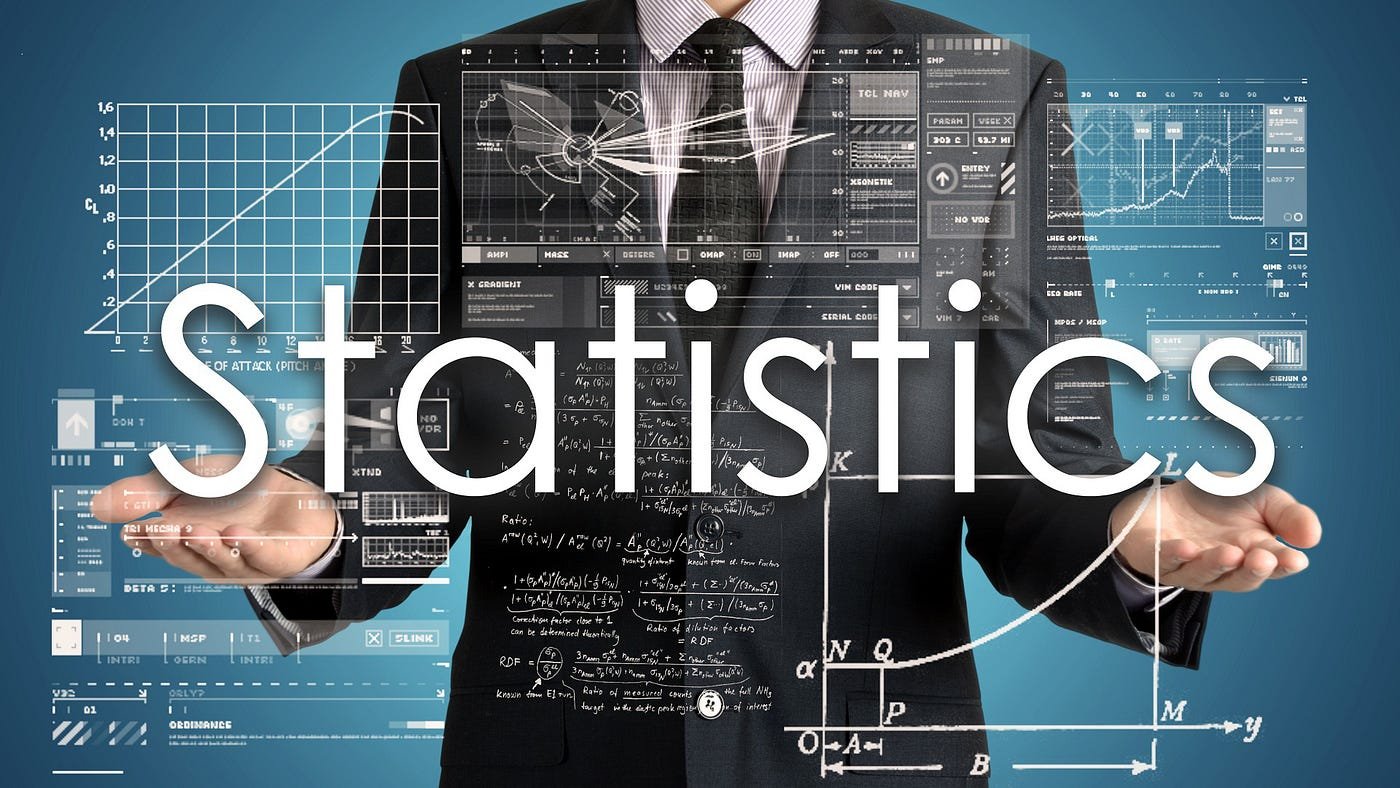
Advantages of Inferential Statistics
- Simplicity: Many methods for different types of data and research questions offer inferential statistics.
- Read more: best 10 latest world news
- Powerful insights: Researchers can see trends and relationships that might not be visible through descriptive statistics alone.
Disadvantages of Inferential statistics
- Sampling error: If the sample does not represent the population, the inference is incorrect.
- Assumptions: Many inferential statistical methods rely on certain assumptions (such as normality) that, if violated, produce results.
- Complexity: Applying and understanding inferential statistics is difficult and requires knowledge of statistical theory.
Read More: The New Startup Revolution: Trends and Strategies for Success- Click Here
Conclusion
Understanding and applying inferential statistics is crucial for researchers and organizations looking to derive meaningful insights from data. By exploring 5 examples of inferential statistics, we see how these methods allow us to make informed predictions, test hypotheses, and generalize findings to a broader population. While inferential statistics has its advantages and disadvantages, its ability to enhance decision-making and predictive analysis makes it an invaluable tool in today’s data-driven world.
Whether you're a researcher, a business professional, or a data enthusiast, mastering inferential statistics can provide you with the skills to navigate the complexities of data analysis effectively.
Other Popular Technology Post:
Object-Oriented Programming | Operating System | Research Methodology | Real Life Integers | Research Hypothesis | Fibonacci Sequence | Exploding Topics of AI | Business Intelligence | Most Searched on YouTube | Finance Topics | One Time Password | Top Google Searches | Research Onion Model | Internal Computer Hardware | Top Social Media Platforms | Scrum Master Roles
Explore other popular Posts:
Blog | News | Entertainment | Education | Sports |
Technology | Cryptocurrency | Stock | Home | Sitemap